
Predictive maintenance is predicting potential machine failures in the factory by analyzing real-time data collected from IoT sensors in machines. Businesses can determine the damage depth, the type of damage, and take action to reduce further damage. Computer vision technology enables businesses to identify damages. In 26 of Amazon’s 175 fulfillment centers, robots help humans for picking, sorting, transporting, and stowing packages.ĭamaged products can lead to unsatisfied customers and churn.
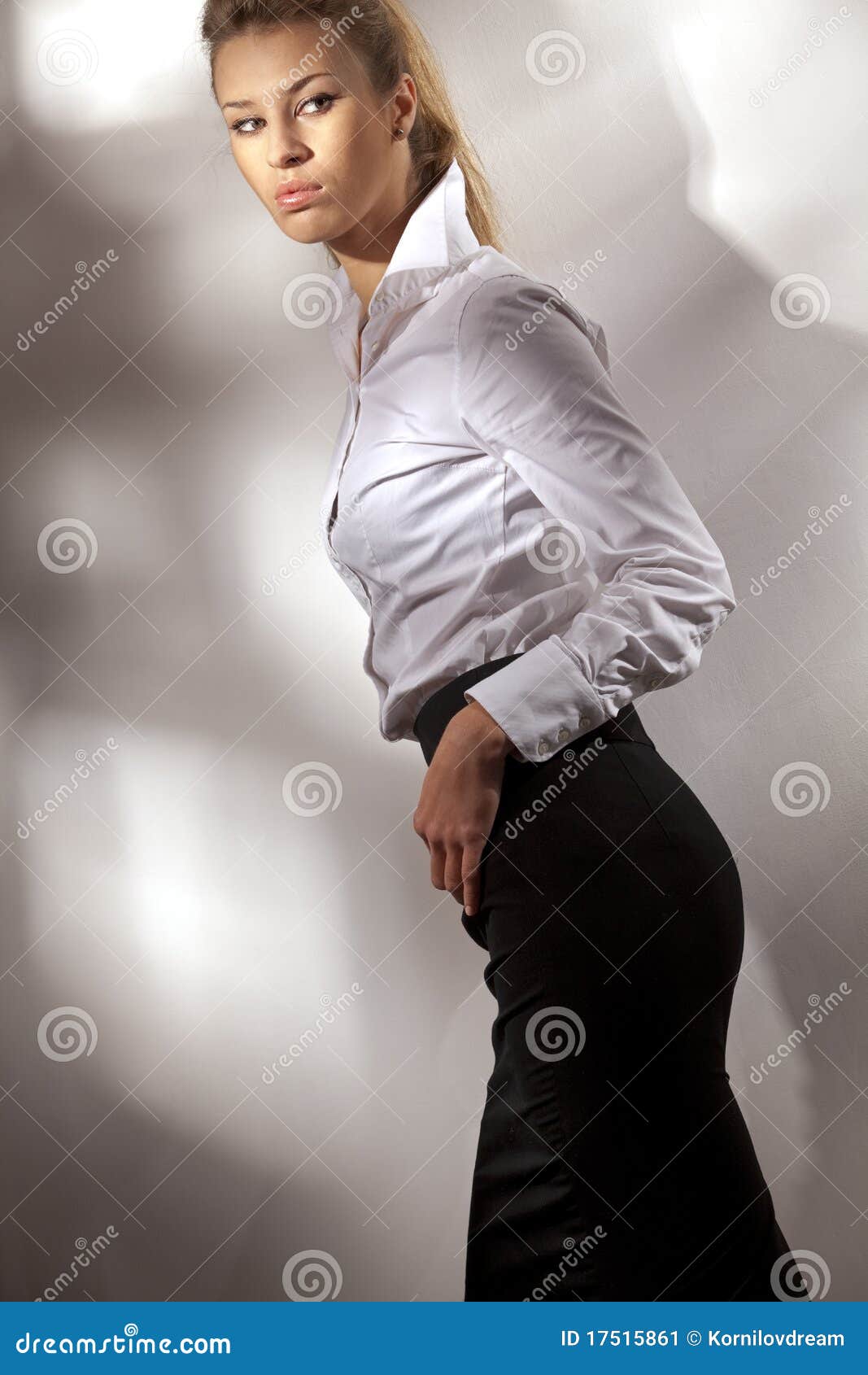
Today, Amazon has 200,000 robots working in their warehouses. The warehouse robotics market was valued at USD 2.28 billion in 2016 and is expected to grow at a CAGR of 11.8% between 20.įor example, the retail giant Amazon has acquired Kiva Systems in 2012 and changed its name to Amazon Robotics in 2015. Warehouse robots are another AI technology that is invested heavily to enhance businesses’ supply chain management. Automated WarehousingĪccording to the 2020 MHI Annual Industry Report, only 12% of businesses are using AI technology in their warehouses, but it is expected to reach 60+% in 6 years. They provide deep learning solutions such as intelligent task automation, demand forecasting, and advanced analytics to retail businesses for enhanced logistics and sustainable manufacturing. Positronic is an AI/ML consultant that helps its clients identify AI/ML use cases and build custom solutions for those use cases. With dynamic supply planning, businesses use fewer resources since dynamic planning minimizes waste.
#History of business intelligence applications update
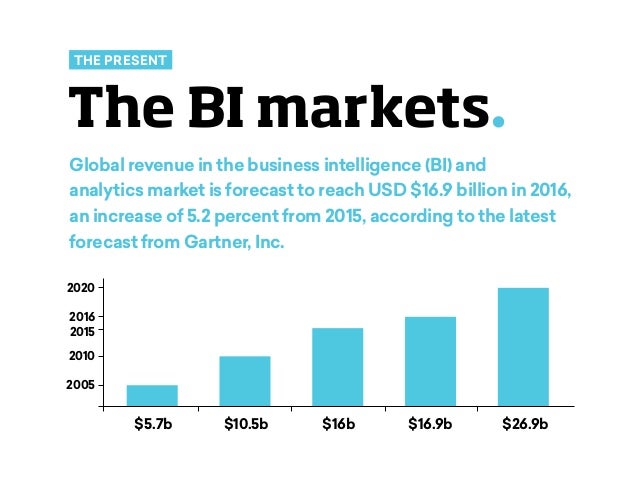

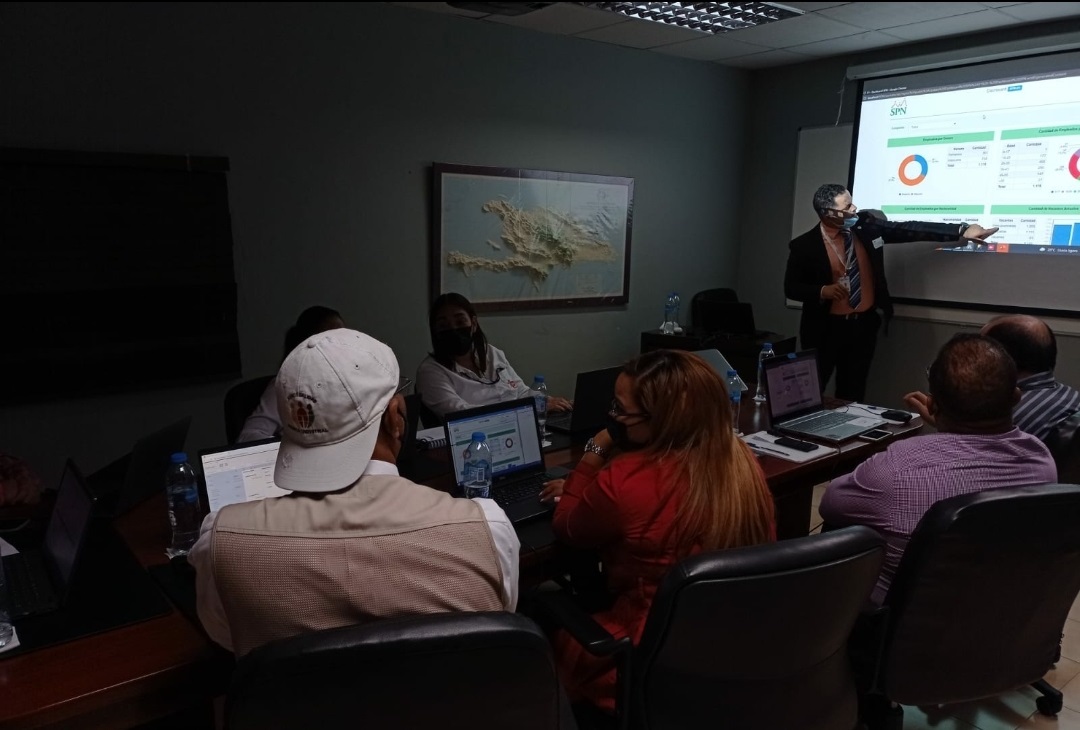
Therefore, AI-powered demand forecasting methods reduce error rates significantly compared to traditional forecasting methods such as ARIMA, AutoRegressive Integrated Moving Average, and exponential smoothing methods. Demand forecastingĪI capabilities enable organizations to use real-time data in their forecasting efforts. Machine learning solutions can facilitate planning activities as they are good at dealing with scenario analysis and numerical analytics, both of which are crucial for planning. Logistics requires significant planning that requires coordinating suppliers, customers and different units within the company. What are the applications of AI in logistics? Planning We will explore top AI use cases in the logistics industry and how they improve logistics operations. According to a McKinsey research, the logistics industry has adopted AI mostly for 4 business functions which cover 87% of AI adoption in logistics:Īnother research by McKinsey estimates that logistics companies will generate $1.3-$2 trillion per year in economic value by adopting AI into their processes.
